Soccer analytics: how data scientists give a new perspective on the game
Published: March 29, 2022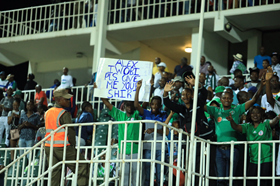
Data analysis is becoming more and more an integral part of soccer every year. For example, not only the coaching skills and innovation of Jurgen Klopp but also the excellent work of the Data Science department under Ian Graham played a significant role in Liverpool's 2018-2020 victories. Graham was able to find common ground with the coaching staff and played a big role in shaping the team and analyzing the game over the last few seasons.
The classic approach, when the match is perceived through the prism of the coach or scout, often misses many things happening on the field and is still a subjective assessment. Data analysis is designed to complement this assessment and provide objective conclusions on each player and the actions of the team as a whole.
According to the professional researcher of edit my paper WritingAPaper, many teams do not make their Data Science departments public. For example, there are 18 teams in the German Bundesliga. And only six of them are known to have at least one Data Scientist. The English Premier League (APL) and Championship League (the two major leagues in England), almost all have such departments. Therefore, how widespread analytics in soccer depends greatly on the country, soccer club, or championship.
Such secrecy is because a detailed analysis of the data gives an advantage to the team in one aspect or another. Just one of the reasons for the success of Liverpool was the development and correct use of mathematical models of the game, ahead of almost all existing at that time. For example, they made a great contribution to their scouting - Liverpool's analysts picked the most suitable players for Klopp's style and principles of play, which helped build one of the best teams of the 2010s.
Data gave Liverpool a competitive advantage a few years ago, and to keep up with the leaders, clubs are allocating more and more budgets to staff. This competitive advantage is extremely important in sports.
What exactly do data scientists and analysts do?
Let's say the analytics department has the task of analyzing the game of the opposing team. To do this they look at the last few games - three or more, depending on the size of the department and the technological solutions available in the club. In the process, the analysts study which tactical formations and game practices were used by the opponent.
When communicating with analysts, the data scientist can be given the task of looking for certain features of the game. For example, to find all the moments when a forward pass is given behind the back or an attack follows a penetrating pass from deep through one of the flanks. Such specified moments are found, saved as XML files, and integrated into the platforms used by analysts for video analysis. This saves analysts a lot of time.
The data scientist may also be asked to find patterns in the actions of players and the team as a whole: construct maps of pass clusters, determine the tactical schemes of the team and their changes during the game, or analyze the decision-making mechanism of the goalkeeper in 1-on-1 situations.
In addition, the data scientist must analyze and implement new metrics that allow a more detailed analysis. In practice, there are too many of them and it would take a separate article to list them all. The simplest and best known is xG (expected goals), which assesses the probability of a goal after a shot. For example, Lionel Messi shoots at an opponent's goal from 25 meters with his left leg at a 45-degree angle, with three defenders surrounding him within 3 meters and the goalkeeper standing 17 meters away. What is the probability that Messi will score a goal?
One of the most advanced metrics in soccer analytics, introduced into soccer from the NBA by the Barcelona analytics department, is the EPV (Expected Possession Value). It is an estimate of whether a team will score the next goal. The estimation takes into account information about the positioning of the players at a given moment. It assumes that a player has three actions that can be accomplished with the ball - to take the ball to a point on the field, pass it, or kick it. Using various mathematical and machine learning models to calculate both the factors that influence the utility of the decision (e.g., the level of pressure applied to the ball carrier) and the probabilities of these three actions, it is estimated whether a team will score a goal or not.
The easiest way to enter the soccer industry right now is through computer vision, which, for soccer, is through object recognition technology on video. Most companies now only give access to two coordinates. That is, the soccer field appears as a two-dimensional object on which points are moving. Of course, it would be interesting to get a third coordinate - how high the ball is flying, what was the position of the player's body in space during the action. This is difficult to implement, and the quality is often very low. Although both Sportec Solutions and Statsbomb still have this third coordinate at least for the ball.
Not long ago, during a soccer match, the artificial intelligence built into the object recognition cameras mistook the referee's bald head for the ball. This resulted in the spectators watching in real-time the entire game, not the movement of the ball on the field, but the referee, who was running a few meters away from what was happening.
The fact is that during the game, the data collection equipment is provided by different companies, and the quality of this equipment is also different. It’s quite the same as with essay writing services. They are all different and provide services of different quality. So, always check subreddit to make sure you choose the high-quality one. In this case, the match was filmed with a very simple Veo camera, which consists of just two lenses: one looks to the left side of the field, the other to the right. Then the camera synchronizes the two images received and a panorama of what is happening is obtained. Since the quality of equipment is worse than that used during major championships, and there were only two cameras, a similar incident occurred.
To compare, in Germany ChyronHego puts 16 to 20 cameras for each stadium served. This amount of equipment ensures a much higher picture accuracy.
How to become a data scientist in the soccer industry
- understand algorithms and technologies already used in the industry;
- look for errors in the data and come up with new/improve old metrics;
- get involved in the soccer community and show as much as possible of your achievements in the analysis of soccer.
It is not necessary to have a degree in programming or Data Science. Coaching experience, even at this level, helps you better understand how players are handled, how to coach them with data analysis in mind, and how a coach can look at and use data.
The classic approach, when the match is perceived through the prism of the coach or scout, often misses many things happening on the field and is still a subjective assessment. Data analysis is designed to complement this assessment and provide objective conclusions on each player and the actions of the team as a whole.
According to the professional researcher of edit my paper WritingAPaper, many teams do not make their Data Science departments public. For example, there are 18 teams in the German Bundesliga. And only six of them are known to have at least one Data Scientist. The English Premier League (APL) and Championship League (the two major leagues in England), almost all have such departments. Therefore, how widespread analytics in soccer depends greatly on the country, soccer club, or championship.
Such secrecy is because a detailed analysis of the data gives an advantage to the team in one aspect or another. Just one of the reasons for the success of Liverpool was the development and correct use of mathematical models of the game, ahead of almost all existing at that time. For example, they made a great contribution to their scouting - Liverpool's analysts picked the most suitable players for Klopp's style and principles of play, which helped build one of the best teams of the 2010s.
Data gave Liverpool a competitive advantage a few years ago, and to keep up with the leaders, clubs are allocating more and more budgets to staff. This competitive advantage is extremely important in sports.
What exactly do data scientists and analysts do?
When communicating with analysts, the data scientist can be given the task of looking for certain features of the game. For example, to find all the moments when a forward pass is given behind the back or an attack follows a penetrating pass from deep through one of the flanks. Such specified moments are found, saved as XML files, and integrated into the platforms used by analysts for video analysis. This saves analysts a lot of time.
The data scientist may also be asked to find patterns in the actions of players and the team as a whole: construct maps of pass clusters, determine the tactical schemes of the team and their changes during the game, or analyze the decision-making mechanism of the goalkeeper in 1-on-1 situations.
In addition, the data scientist must analyze and implement new metrics that allow a more detailed analysis. In practice, there are too many of them and it would take a separate article to list them all. The simplest and best known is xG (expected goals), which assesses the probability of a goal after a shot. For example, Lionel Messi shoots at an opponent's goal from 25 meters with his left leg at a 45-degree angle, with three defenders surrounding him within 3 meters and the goalkeeper standing 17 meters away. What is the probability that Messi will score a goal?
One of the most advanced metrics in soccer analytics, introduced into soccer from the NBA by the Barcelona analytics department, is the EPV (Expected Possession Value). It is an estimate of whether a team will score the next goal. The estimation takes into account information about the positioning of the players at a given moment. It assumes that a player has three actions that can be accomplished with the ball - to take the ball to a point on the field, pass it, or kick it. Using various mathematical and machine learning models to calculate both the factors that influence the utility of the decision (e.g., the level of pressure applied to the ball carrier) and the probabilities of these three actions, it is estimated whether a team will score a goal or not.
A bald head instead of a ball or why artificial intelligence is not omnipotent
Not long ago, during a soccer match, the artificial intelligence built into the object recognition cameras mistook the referee's bald head for the ball. This resulted in the spectators watching in real-time the entire game, not the movement of the ball on the field, but the referee, who was running a few meters away from what was happening.
The fact is that during the game, the data collection equipment is provided by different companies, and the quality of this equipment is also different. It’s quite the same as with essay writing services. They are all different and provide services of different quality. So, always check subreddit to make sure you choose the high-quality one. In this case, the match was filmed with a very simple Veo camera, which consists of just two lenses: one looks to the left side of the field, the other to the right. Then the camera synchronizes the two images received and a panorama of what is happening is obtained. Since the quality of equipment is worse than that used during major championships, and there were only two cameras, a similar incident occurred.
To compare, in Germany ChyronHego puts 16 to 20 cameras for each stadium served. This amount of equipment ensures a much higher picture accuracy.
How to become a data scientist in the soccer industry
To enter the soccer industry as a data scientist, you first need to have certain skills:
- be able to code and visualize data;- understand algorithms and technologies already used in the industry;
- look for errors in the data and come up with new/improve old metrics;
- get involved in the soccer community and show as much as possible of your achievements in the analysis of soccer.
It is not necessary to have a degree in programming or Data Science. Coaching experience, even at this level, helps you better understand how players are handled, how to coach them with data analysis in mind, and how a coach can look at and use data.
Latest News
- No word from Nigeria: Eric Chelle yet to reach out to Arsenal midfielder Nwaneri ahead of U21 Euro
- 'I was childish' - Iwobi finally speaks on social media post celebrating Super Eagles call-up in 2021
- Flying Eagles centre-back wins league title with Brentford U21s
- 'His girlfriend wants him to stay' - Insider makes bold claim about Galatasaray striker Osimhen
- 'A great one' - Ilechukwu hails Chelle for calling up NPFL stars, urges home-based players to dream big
- Following Super Eagles players in international tournaments
- 'One of the best' - Super Eagles assistant coach raves about Rangers midfielder after Unity Cup call-up
- 'We haven't heard' - Werder Bremen chief responds to Felix Agu's links with Fulham, West Ham
- 'I'll never forget' - Super Eagles defender Semi Ajayi breaks silence after release by West Brom
- Winners medals: Nigerian teenagers pictured with Spurs captain after Europa League triumph
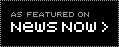
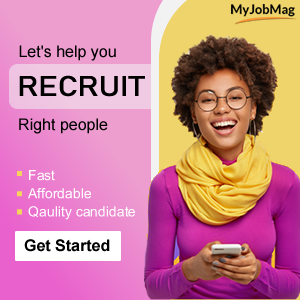
News Categories
- Football Betting
- African Players
- Australia/ Asia
- Belgian Championship
- Bundesliga
- Danish Football
- English Premier League
- Nigeria National Teams
- Casinos not on Gamstop
- Nigeria Premier League
- Norwegian Football
- Polish Football
- Russian Championship
- Serie A
- Spanish Liga
- Swedish Championship
- Transfer
- Turkish Championship
- 1Win
- Ukrainian Football
- Uncategorized
- Women's Football
- keo nha cai
- F168
- hi88
- hi88
- hi88
- hi88